Why Machine Learning Is Great Elly For Cyber Security.
Although we have spoken on several occasions about Machine Learning and even our Augur product , this time we will do a little theoretical review for those who are not so familiar with this technology.
Table of Contents
What is Machine Learning?
The machine learning , English Machine Learning (ML), is a branch of science that allows computers through a set of techniques to perform tasks without being explicitly programmed. Through ML, computers can generalize their behavior from processed data in order to make predictions about future data.
By way of context, the term Machine Learning has existed for several decades, when Arthur Samuel used it for the first time in IBM laboratories in 1959 and defined it as:
“Field of study that gives computers the ability to learn without being explicitly programmed”
However, it was only in the 1980s when this concept gained more force with the appearance of artificial neural networks (ANN – Artificial Neural Network) and then after another decade it began to be used by various specialists with the aim of solving some problems of daily life.
Similar to what happened in early 2010 with Cloud technologies when many considered that they were not going to take hold, the same happened with ML. Today this science is used by various companies: Facebook, Netflix, YouTube, Google or Amazon, to name a few.
The most popular systems that use Machine Learning are voice recognition and facial recognition, customer profiling in marketing, market studies, and the latter is being added automation for IoT, autonomous cars, and even the famous aid robots. .
Now, the central question is: what kind of needs could Machine Learning satisfy in the cybersecurity industry? To answer this, we must first give a small theoretical framework to understand where we could apply Machine Learning in cybersecurity.
How is ML classified in general?
Broadly speaking, we can classify it as:
Supervised learning: it is focused on determining the probabilities of new events based on previously observed events. Within this algorithm we find two other categories:
- Classification: Classification algorithms predict which category an entry belongs to based on the learned probabilities of previously observed entries. For example: determining if a file is malware or not.
- Regression: Regression models (linear, logistic) predict an output value for a given input based on the output values associated with the previous inputs. For example: predicting how many malware samples will be detected in the next month.
Unsupervised learning: they try to find unlabeled patterns. For example: determining how many malware families exist in the dataset and which files belong to each family. Within this type of ML is “ Clustering ”, which consists of grouping a set of objects (cluster) by their similarities. Example: detection of anomalies, or malware families.
Stages of Machine Learning
Even if you are not an expert in this type of technology, it is important to understand in general terms how the general ML process works, which is divided into the following stages:
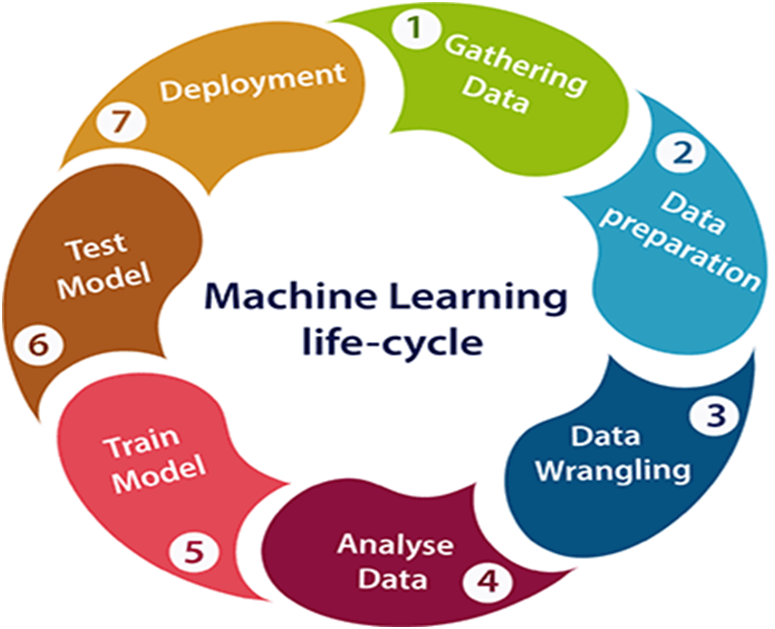
- Whatever the ML algorithm to use, you must have a large number of data to train our model. Most of the data comes from various sources.
- many times the data collected is categorical, so it is necessary to perform a preprocessing and transform that data into numeric, since ML algorithms work only with numerical data.
- Items to be extracted and analyzed are identified.
- the attributes necessary to train the ML model are identified.
- : the model is trained based on the selected ML algorithm. In this stage, part of the data is used to train the model and another part to evaluate it.
- : it is considered by many experts the most important stage, since, having the model trained, the model must be validated. For this, the data that was separated in the previous stage, validation data, are used to run the ML model and evaluate if the model offers the expected results.
- in this stage the errors to correct and adjust the model are searched.
Having already explained the types of ML that exist and their stages, we will proceed to detail the areas where this technology could be used within cybersecurity.
Cybersecurity areas in which Machine Learning is being applied
Generally, machine learning products are built to predict attacks before they occur, but given the sophisticated nature of these attacks, preventive measures often fail. In such cases, machine learning helps remedy in other ways how to recognize the attack in its early stages and prevent it from spreading throughout the organization. The following figure identifies the needs that ML could cover within the field of cybersecurity:
The B-side of Machine Learning
For now, we only talk about how Machine Learning could become an ally for the cybersecurity field, but we must not forget that ML is currently used for various areas. For example: facial recognition, in the field of genetics, text compression, autonomous vehicles and robots, image analysis, fraud detection, predicting traffic, customer selection, search engine positioning, voice recognition, among other applications. However, all these types of applications work from the processing of huge amounts of data.
The question then is: Can a Machine Learning model be compromised by cybercriminals? The answer is yes.
Just as prevention models for different technologies are studied within cybersecurity, currently it is beginning to focus on the application of ML to these models. For this reason, within the field of cybersecurity, the concept of Adversarial Machine Learning is gaining more relevance.
What is Adversarial Machine Learning?
The term “adversary” is used in the field of cybersecurity to describe the procedure by which an attempt is made to penetrate or corrupt a network.
n this case, adversaries can use a variety of attack methods to disrupt a machine learning model, either during the training phase (called a poisoning attack) or after the classifier has been trained (a attack of “evasion”).
Conclusion
In recent years the term Machine Learning has taken on more importance for systems, of course it is a type of technology that is growing and has many benefits for various sectors. Regarding the cybersecurity area, ML can be used in Threat Intelligence ; for example, in the detection of threats, since this area produces a large volume of data at its start.
In addition, it is seeking to include it within the Threat Hunting areas and for the accurate classification of malware families.
Undoubtedly, this technology is a great ally for multiple sectors, but there is the possibility that these data intelligence models are modified and that this seriously affects the business that is using them. In a future article we will delve into the subject of Adversarial Machine Learning.